3-part Podcast Series Presented by Eularis with Industry Experts
Artificial Intelligence is evolving from being an important strategic investment to a must-have at scale today for pharmaceutical companies.
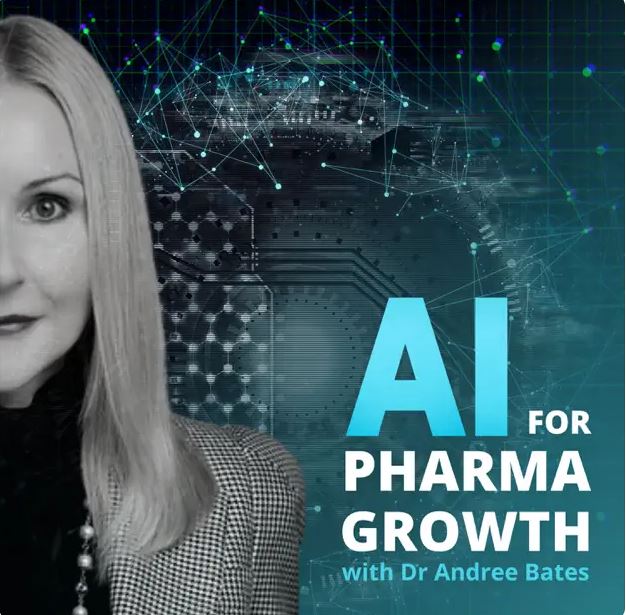
More specifically, much of the pharmaceutical industry is introducing artificial intelligence powered next best action (NBA) to drive proactive business intelligence into commercial execution. NBA, also known as context marketing, identifies the needs of individual physicians at specific moments of engagement to assist a life science organization in delivering the right personalized content to the right channels, at the right time.
This personalized and tailored engagement leads to a much enhanced customer experience – ultimately, delivering stronger business results.
While there is solid consensus that AI, and specifically NBA, are essential for long-term growth and success in life sciences organizations, it is still a dynamically evolving landscape – with much to learn from pioneers and early adopters.
Dr. Andree Bates, founder of Eularis, and host of the AI for Pharma Growth Podcast, explores the challenges and opportunities with delivering NBA at scale in a 3-part podcast series with guests Olivier Aurand, IT Director at Mundipharma and Alan Kalton, Senior Vice President of Global Sales at Aktana.
Olivier Aurand shares the point of view of a major pharmaceutical company about how to make good decisions while introducing NBA within the organization. Mundipharma, an early adopter of NBA, is a global leader in pain and supportive care with $2 billion in annual revenue.
Pharma next-best-action initiatives present “unique opportunities and sometimes the first opportunity to bring marketers and sales teams together,” says Aurand. “Where prior to that, they may not always have worked in a sufficiently integrated manner.”
In contrast, Alan Kalton, provides the view of Aktana, a pioneer in NBA solutions, in how customer needs are evolving, and offers best practice insights for delivering AI at scale.
“This is, as a technology capability for the pharmaceutical industry, moved very substantially from an investment as a strategic bright light four or five years ago, to now an investment that needs to be considered at scale,” says Kalton.
For key insights about choosing and implementing Next-Best-Action, listen to the pharma perspective and the vendor perspective on the following topics:
Episode 1: The Data Conundrum
Episode 2: How to Design NBA Systems for Global Scale in Pharma
Episode 3: How to Define NBA Implementation Success in Pharma
Here are some highlights shared by Aurand and Kalton in the 3-part series:
Next best action requires collecting volumes of data, but do you invest in the data first? Or do you create ways to stimulate behavioral change and improve your understanding of the impact?
Olivier Aurand with the pharma perspective: Both are required, and I think it’s about looking at how you can do that on a smaller scale initially, maybe considering pilots. Whether they are pilots in terms of granting certain application capabilities, and not trying to boil the ocean uncovering all the capabilities at the same time.
Alan Kalton with the vendor perspective: There’s a whole pattern here from putting in the right infrastructure, acquiring the right information, performing the right analytics, and then activating those analytics to drive behavioral change.
You want to experiment and you want to explore, to get to socialization as quickly as possible – because not only does that drive the end result, but it can also provide a frame for how much you invest going forward.
And how do you prioritize data versus stimulating behavior?
Aurand: The scale of the investments required, versus the perceived risk to achieve the expected outcomes can be scary. I think taking baby steps and having a lower financial risk and investments initially, demonstrating the value, for me is a very good tactic. That way, we can reassure the organization, and if done right, can help in securing the funding required to take it to the next level.
Kalton: And so as you’re building a program, focusing on an ability to get time to value, getting that experience, and building the business case, doing so as a live approach is becoming more and more important.
How can pharma determine readiness of their data?
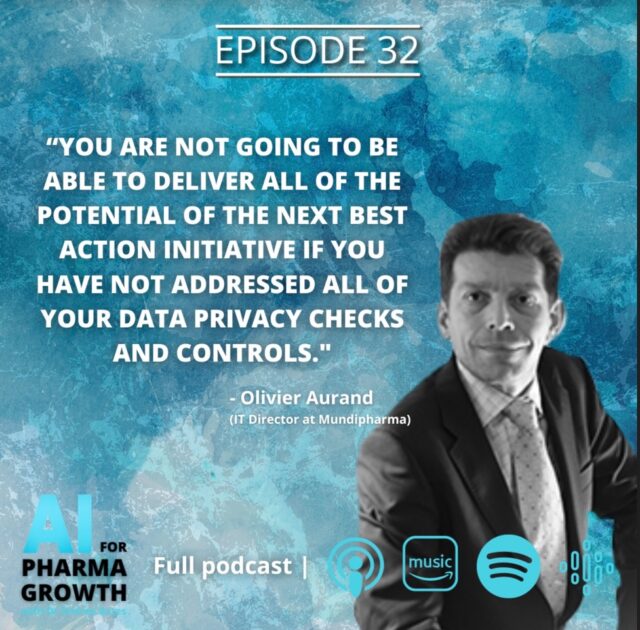
Aurand: Even if you can define readiness, achieving 100% readiness is extremely difficult. I think it is important to start with what you’ve got. It might be more directional, rather than very precise. But it’s important to keep moving forward.
Kalton: We help our clients define what readiness means. And what it really is about is being ready for the journey, and ready for that behavioral change. Within the patchwork of data that exists within life sciences companies, there’s always going to be something valuable that’s ready to stimulate behavioral change. It’s about understanding the journey and how to build that confidence and those experiences over time.
What are emerging challenges with deploying pharma next-best-action systems to support a scaled approach?
Aurand: Most pharma companies have done a good job at being content factories and trying to develop content once to reuse in different markets. But as you go omnichannel and drive NBA, the ability to create content or micro content and localize while still meeting all of the regulatory requirements in each of the markets is something not to be underestimated.
Kalton: There are unique data environments, data sources and regulatory environments. And there are also unique ways in which pharma organizations work that may be different from market to market. So the ability to connect that through an underpinning technology to inspire the right behavioral change in individual markets is a critical next step.
The ability to create levels of self engagement with tuning at a local level is much more cost efficient and creates that level of local ownership. I think it’s a really, really important dimension of where this technology is going in the marketplace.
What is your advice to pharma leaders who want to engage their organizations in designing Next-Best-Action programs at scale?
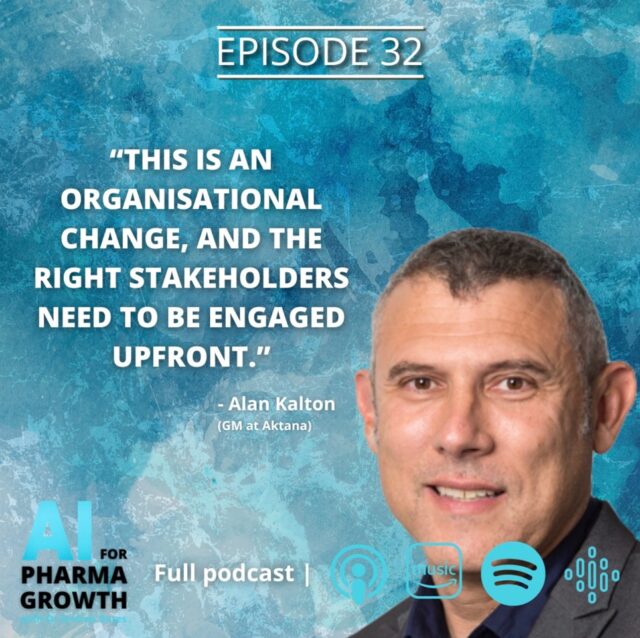
Aurand: I would say align on the high level direction. Then start small and demonstrate value often, as quickly as you can, even if it is small value at the beginning. Focus on selling the benefits of those capabilities. Think about all of the stakeholders who will be involved, be impacted and benefit from the value. And take a bigger pace over time, while you learn as you go.
Kalton: It’s about the organization, recognizing this is an organizational change process and the right stakeholders need to be engaged upfront. And that means stakeholders across the complete organization, whether they’re part of the regulatory function, whether they’re part of the medical function, whether they are part of commercial or technology, it is a combined play that’s necessary to be successful.
What does successful NBA implementation look like?
Aurand: Such an initiative can only be successful with cross functionality. Everybody contributes and we all understand the challenges and opportunities, from a sales and marketing perspective, medical perspective and legal compliance. I’m amazed with how this has enabled us to further improve understanding of each of the functions and the collaboration. It’s been an amazing journey as part of our overall transformation here at a big pharma.
Kalton: I’ll lay out a little bit of a framework which is centered on the fundamental focus of this being a behavioral change process. And as you go through the steps towards that behavioral change, you look to gain an understanding of how well people are engaging with the change management and with the technology that’s introduced to support this. One of the fundamental pieces to that engagement is how well you execute. So part of NBA as a concept is accelerating your ability to control execution of strategy.
But by inherently doing this from a corporate perspective, you’ve created agility. Because you can adapt and change your strategy, you no longer have to wait for the next annual planning cycle, or the next quarterly meeting to roll out new targets. You can adjust an algorithm or just a methodology in real-time and refine your strategy.
Interested in seeing if your organization is ready to implement an AI-driven customer engagement solution? Request a Data Readiness Assessment from us.