Derek Choy on Trust: Why Transparency in Agentic AI Matters for Life Sciences
A pivotal lesson from our early AI implementations is that transparency is non-negotiable. In one of our earliest deployments with a major pharma company, we observed firsthand how skepticism arose when AI recommendations felt like unexplained commands rather than thoughtful suggestions. Field representatives questioned the value of AI-driven recommendations because they lacked clear, relatable reasoning. This was understandable—after all, these representatives had significant experience and relied on their judgment and deep customer relationships. For them, trust in AI-driven recommendations was driven not just by accuracy but also intuitive, relatable context that “felt right” in the light of their own professional insights.
The turning point came when we started providing transparent, contextual reasons based on relevant, real-world data for every AI recommendation. This included context like recent HCP interactions, historical prescribing patterns, peer comparison insights, and even subtle indicators like the HCP’s preferred communication channel and timing. This made AI recommendations feel actionable and logical to reps, increasing adoption and confidence in the system.
Transparency in Agentic AI is also crucial for strategic brand management. When brand leaders can clearly trace the reasoning behind AI-driven strategies, they can more confidently delegate strategic decisions to semi-automated or even fully-automated systems. This enables brand managers to quickly validate, adjust, or embrace recommended strategies without extensive manual review.
Today, Aktana’s Agentic AI approach institutionalizes this principle of transparency. Every recommendation our system provides is fully traceable and understandable, ensuring compliance with strict regulatory standards and also meaningful human oversight. Our central orchestration engine serves as a transparency hub, providing clear visibility into every decision: why it was recommended, what data informed it, and how it aligns with strategic goals. This explainability is vital for compliance teams, ensuring complete auditability to satisfy stringent regulatory requirements.
Ultimately, transparency and traceability are essential for both building trust and also maintaining sustained confidence and adoption. By embedding these qualities into our Agentic AI platform, we empower decision-makers with clarity, control, and trust—paving the way for the scalable, reliable omnichannel orchestration essential to the success of today’s pharma organizations.
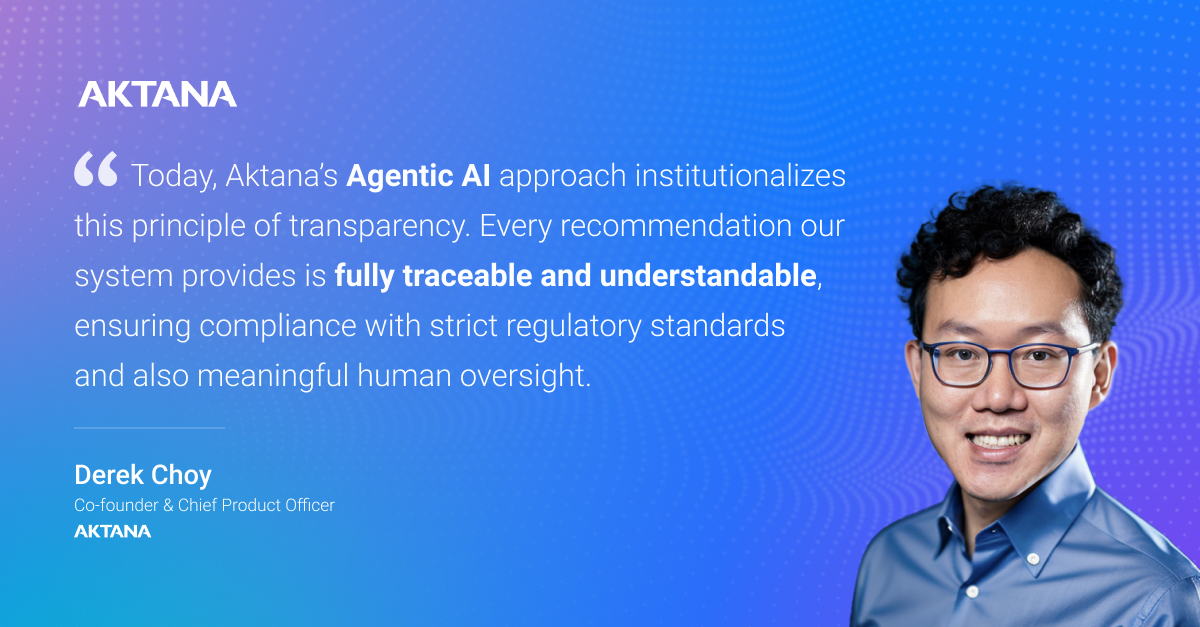
The FAQs below address how Agentic AI earns trust—by making every decision explainable, auditable, and aligned with strategy. If you’re wondering how transparency in Agentic AI works in practice, start here.
Frequently Asked Questions on Agentic AI
In a regulated, data-intensive industry like life sciences, autonomous AI agents have the potential to significantly improve how organizations engage, operate, and scale—if designed and deployed with care. The following examples highlight the kinds of benefits that a well-architected Agentic AI system could deliver:
1. Dynamic Communication Planning and Personalization
Agents can now leverage AI to craft and deliver highly personalized and contextually resonant communication plans. They can dynamically adapt messages, channels, and timing in real time based on detailed HCP behavior, brand objectives, and engagement patterns. This can result in communications that truly speak to each individual, maximizing impact, improving content utilization, and freeing up field teams to focus on deeper relationships.
2. Coordinated Execution Across Channels and Teams
Multiple agents can operate in parallel to orchestrate omnichannel strategies, monitor field capacity, adjust next-best actions, and enforce brand priorities. This, in turn, enables faster campaign execution and cross-functional alignment without sacrificing control.
3. Transparent, Auditable Decision-Making
Every recommendation from the system should come with clear reasoning—from content suggestions to tactical adjustments. This supports explainability, builds trust across teams, and ensures decisions are easy to validate and audit.
4. Adaptation Without Disruption
Agents will be able to autonomously respond to market and regulatory changes such as new product launches or label updates. By eliminating manual reconfiguration, an agentic system should remain agile even during periods of transition.
A multi-agent decision ecosystem enables AI agents to process data, apply expertise, and act autonomously in real time. This foundation for practical Agentic AI ensures transparency, human oversight, and alignment with strategy and regulations. Such architecture powers platforms like Aktana’s, enabling scalable, trusted automation.
How this works in practice:
This visual demonstrates the collaborative nature of an illustrative multi-agent decision ecosystem, showcasing how a wide range of specialist agents can work together to enable personalized communication, strategic execution, and transparent decision-making at scale.
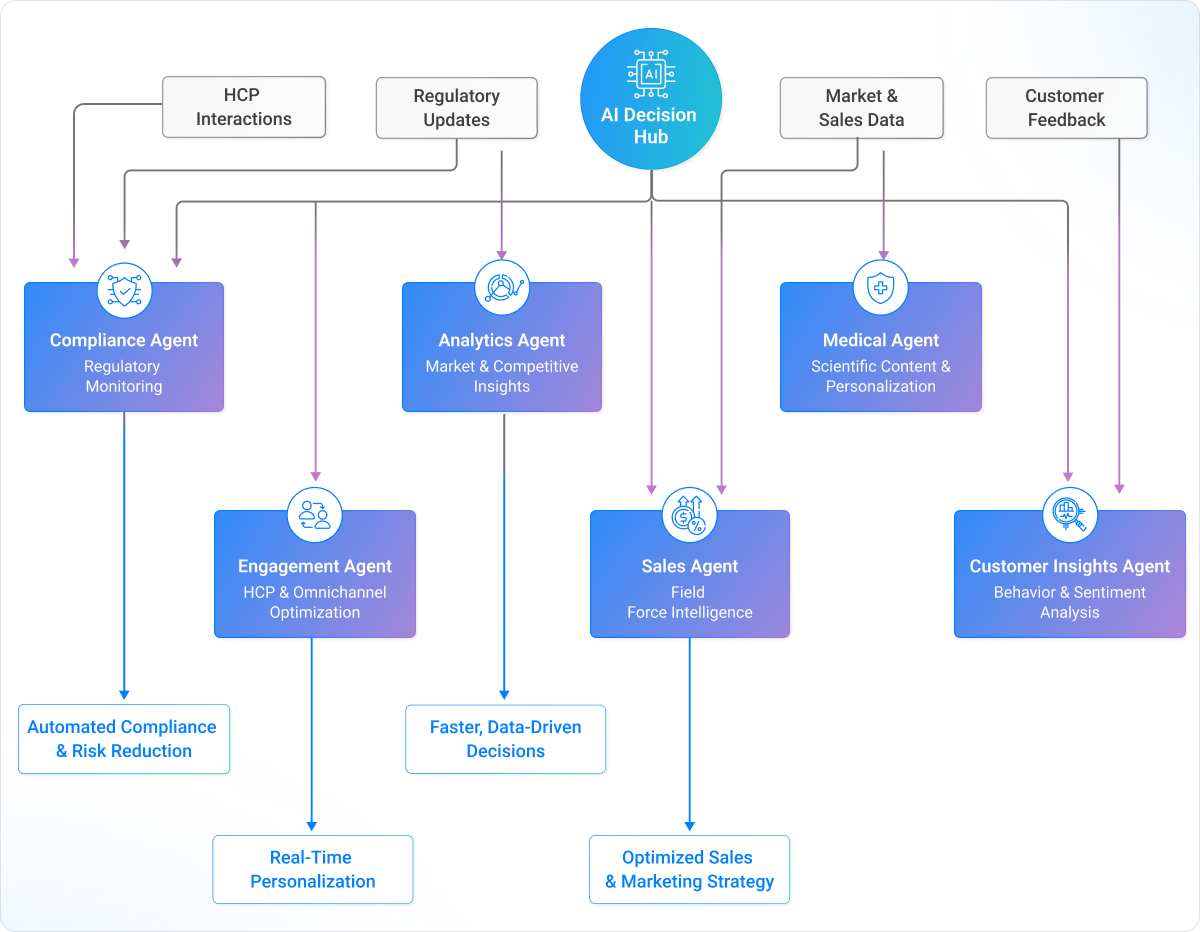
While both Generative AI and Agentic AI advance artificial intelligence, they address distinct needs through different approaches.
- Generative AI focuses on content creation—generating text, images, or code—by identifying patterns in data. It automates content creation and summarizes information but does not make independent operational decisions.
- Agentic AI, on the other hand, expands the utility of Generative AI but is much more geared towards action and orchestration. It plans workflows, executes strategies in real-time, and coordinates tasks. It functions as a collaborative ecosystem of AI agents working together to optimize operations and ensure compliance.
In short, Generative AI enhances content production, while Agentic AI drives complex operations. Crucially, Agentic AI can utilize Generative AI outputs to craft highly personalized, nuanced communications, such as tailored emails, dynamic summaries, and context-specific content variations, and strategically deploy them in real time based on objectives and HCP responsiveness.
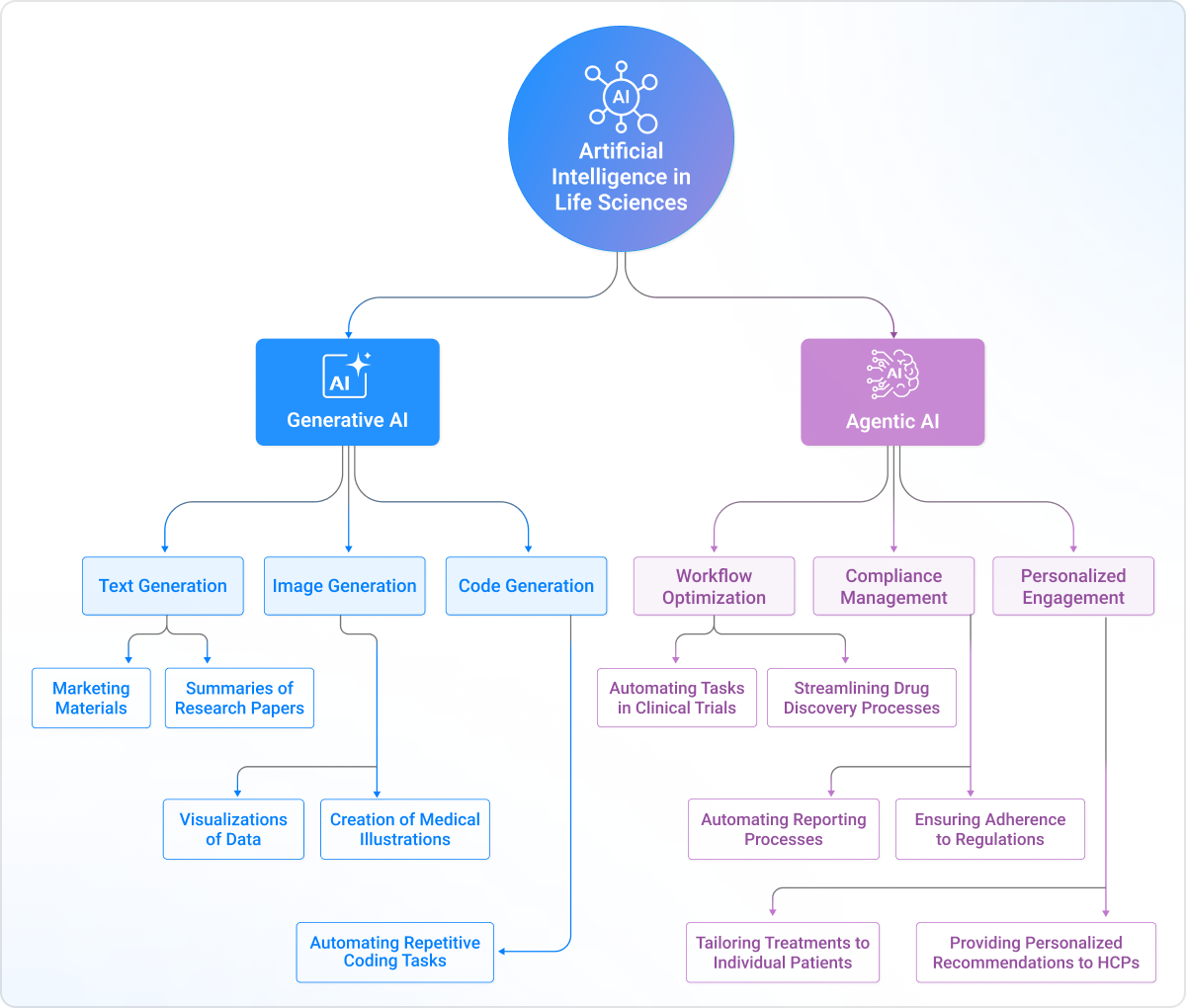
Trust is paramount in life sciences, where decisions impact compliance, patient safety, brand integrity, and field adoption. “Black box” AI, which provides outputs without explaining their origins, presents significant risks:
- Field teams may dismiss recommendations they cannot understand.
- Brand leaders may struggle to validate AI-driven strategies.
- Regulatory teams may be unable to audit or defend decisions.
Well-designed and high-quality Agentic AI, if approached in the right way, can mitigate these risks with transparent, explainable reasoning, providing context such as HCP engagement data, prescribing patterns, and constraints for each recommendation. This is why separating out the agent which transparency orchestrates and optimizes is paramount. This inherent traceability allows brand teams to ensure strategic alignment, compliance officers to track a complete audit trail, and field users to trust suggestions as both accurate and logical.
Explainability in AI means understanding how and why an AI system makes a recommendation, fostering trust and building confidence. ‘Black box’ AI breeds skepticism.
Here’s why explainability is critical for AI adoption:
- Builds Confidence: Understanding the reasoning behind AI recommendations—the data, logic, and “why”—gives teams confidence to act, transforming AI into a trusted partner.
- Validates Team Expertise: Explainable AI aligns with field team experience, providing context that supports their judgment. This is particularly crucial when AI selects or sequences content. When reps and brand managers understand why a particular personalized message or communication arc was chosen—based on strategy, engagement, or behavioral signals—they are more likely to trust and use the system.
- Ensures Accountability: Transparency allows teams to validate, track, and ensure compliance. Understanding is key to accountability.
- Facilitates Adoption: When AI’s decisions are clear, it is more likely to be used. Explainability removes barriers to adoption.
Ultimately, explainability empowers teams, making AI a trusted tool that turns data into actionable insights.
In life sciences, even a small error, like incorrect dosage information in AI-generated content, can trigger product recalls, regulatory investigations, and reputational damage. That is why, for Agentic AI to succeed in this space, compliance cannot be an add-on; it must be foundational.
From the outset, all AI-driven actions should be governed by clear business rules and subject to human oversight. These rules serve as guardrails, ensuring that even as agents operate autonomously, they never deviate from approved processes or content.
To further mitigate risk, Agentic AI systems must undergo rigorous testing—evaluated against a wide range of simulated scenarios, edge cases, and adversarial prompts. This ensures the system performs reliably under regulatory pressure, even in complex or unexpected conditions.
Transparency is equally essential. Like a detailed patient record, every AI decision must be fully traceable: what the system did, why it did it, and whether it stayed within regulatory boundaries. Tools like MLflow in Databricks help log and monitor these decisions, creating a defensible audit trail that supports compliance reviews and internal governance.
Embedding compliance, rigorous testing, and full transparency enables life sciences organizations to confidently innovate while meeting industry standards for speed and scale. This is especially important in content-driven workflows, where AI agents are responsible for not just delivering—but also selecting—appropriate, pre-approved messages and sequencing them within regulatory boundaries. Every step must be explainable, auditable, and compliant.
Agentic AI’s strengths in synthesis, planning, and action make it ideal for near real-time applications like pre-call planning, route optimization and customer agent interactions — what we might think of as ‘last mile, local orchestration.’ However, for use cases like end-to-end intelligent omnichannel engagement in high-stakes and heavily-regulated fields like life sciences, relying solely on these capabilities poses significant risks. Without a centralized orchestration layer that includes a way to capture strategy and goals, and a built-in knowledge base, autonomous agents could make decisions that are misaligned with strategic goals, lack transparency, and ultimately compromise leadership oversight.
This highlights the critical roles of Aktana’s Optimizer, Orchestrator and Knowledge Nexus.
Aktana’s Optimizer & Orchestrator, function as the centralized intelligence layer that guides Agentic AI, transcending a simple decision engine. Driven by a Dynamically-Constrained Optimization Engine, Aktana calibrates every action in real-time across channels, constraints, and competing priorities and ensures that ROI is always maximized.
The result is a Scored Forecast, assigning economic value to each potential omnichannel action. This guarantees that agentic recommendations are proactive but also thoroughly informed, ultimately driving higher-impact business outcomes rather than perpetuating ineffective past strategies.
Aktana’s Knowledge Nexus, is crucial for Agentic AI, as it provides the necessary context from over a decade of life sciences best practice and experience. With 5,000+ proven tactics and 85+ validated use cases, it grounds every AI-driven action in real-world success – providing ready-to-use blueprints for boosting tactic effectiveness, accelerating impact, and maximizing ROI. This knowledge base ensures AI agents operate within a structured framework, learn from past strategies, and optimize performance using benchmark data.
Why This Matters For Life Sciences
Trust is fundamental in life sciences. To ensure that AI decisions are transparent, compliant, and strategically robust, Agentic AI requires integration with a sophisticated intelligence layer. Solutions like Aktana’s Optimizer, Orchestrator, and Knowledge Nexus are vital in this regard. We believe that only through such a synergistic combination—where autonomous execution is guided by learned intelligence, contextual knowledge, and global optimization—can AI truly drive transformative, industry-wide advancements
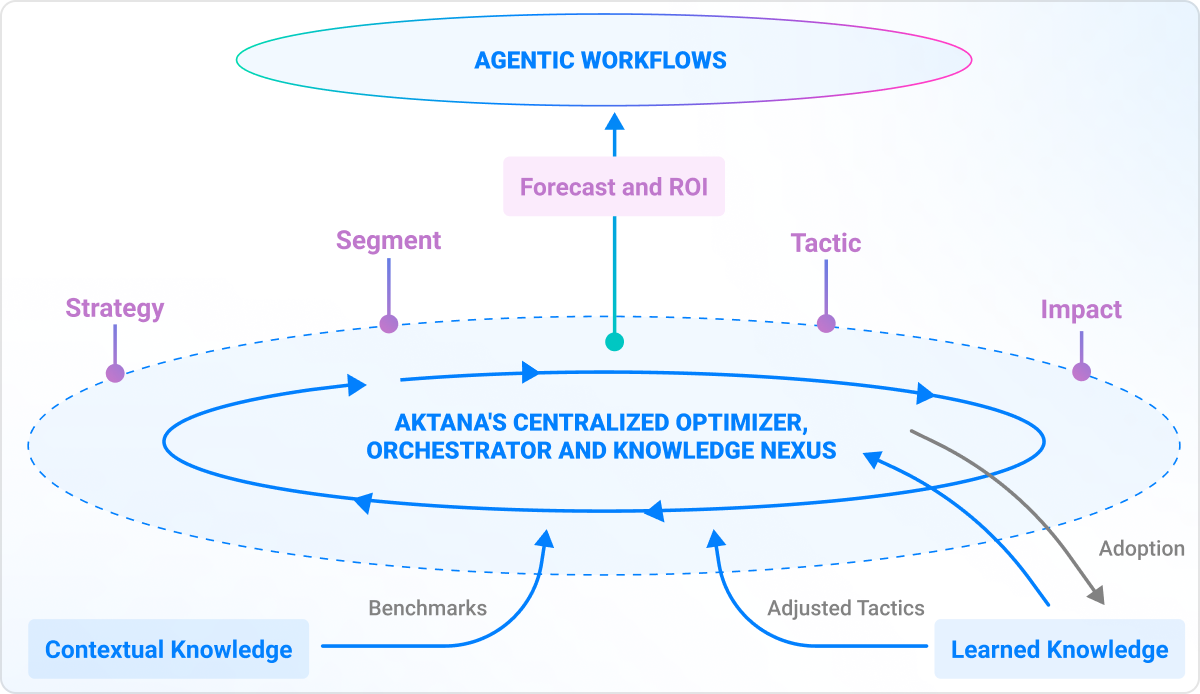
Imagine a world where slow, siloed processes and endless manual reviews no longer hold your teams back. That’s the promise of Agentic AI: replacing fragmented workflows with intelligent, adaptive systems that operate in real time. This includes the ability to dynamically create and deliver personalized communications that resonate with each healthcare professional.
- From Manual to Automated Compliance
Agentic AI goes beyond simply checking for errors—it actively tailors communications to specific needs and preferences while ensuring continuous compliance.
➡ A marketing team can now utilize AI to craft diverse, personalized assets that are instantly vetted against business rules, accelerating time to market with communications that are both engaging and fully compliant.
- From Reactive to Proactive Decision-Making
Rather than merely responding to lagging indicators, AI can provide near real-time intelligence for all aspects of operations– from clinical trial operations to engagement strategy.
➡ AI could analyze real-time patient data and trial progress to recommend protocol optimizations, thereby shortening trial cycles and accelerating the launch of new brands or indications.
➡ Similarly, if prescribing behavior shifts, field priorities adjust immediately—no need to wait for end-of-quarter analysis.
- From Siloed to Connected Teams
Medical, commercial, and regulatory teams often work in parallel, not in sync. Agentic AI connects teams by automatically routing insights and approvals, fostering cross-functional coordination.
➡ HCP questions are triaged to the correct expert instantly, improving both response speed and accuracy.
- From Static to Adaptive Resource Allocation
Conventional planning is based on historical assumptions. An Agentic AI system can dynamically reallocate resources based on live data.
➡ When competitor activity spikes in a particular region, sales efforts shift accordingly—maximizing impact without added headcount.
Agentic AI transforms automation. By embedding intelligence into every layer of the operation, it can help life sciences shift from reactive execution to strategic coordination, freeing teams to deliver more value.
See the potential in action: The diagram below illustrates how these elements connect. You’ll see how Agentic AI processes real-world inputs like regulatory updates, HCP interactions, and patient data to drive decisions and execute actions, all while continuously learning and improving.
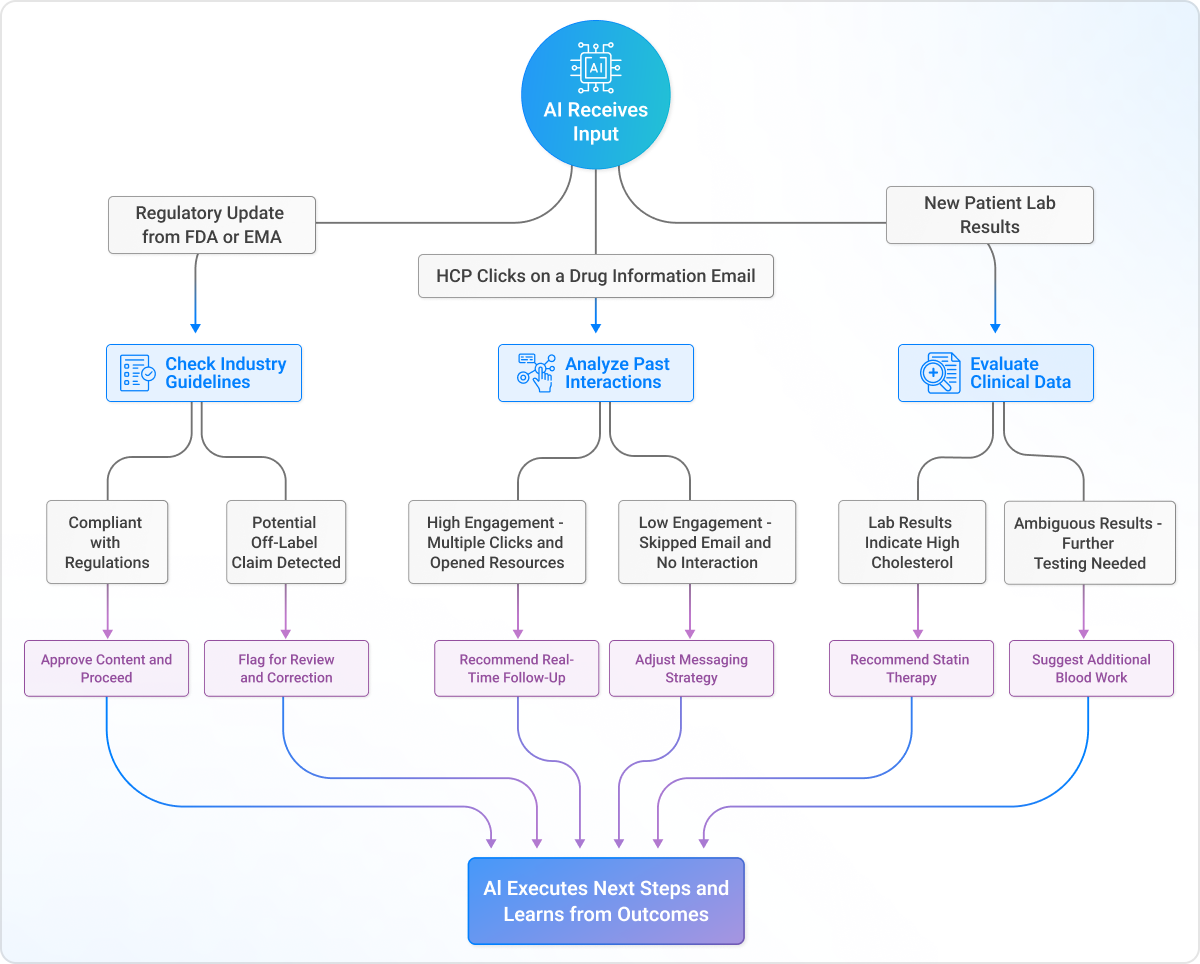
What’s Coming Up Next
In the final part of this series, we’ll move beyond trust to explore what makes Agentic AI truly effective—from real-time learning to practical orchestration. We’ll show how life sciences teams can build toward autonomy without losing control.
In the meantime, explore the first part in this series to see how human expertise gives Agentic AI its edge—or visit our Agentic AI page to discover how these principles power real-world use cases in life sciences, and why industry leaders are rethinking what AI can truly deliver.