From the Desk of Derek Choy: A Practical Vision for Agentic AI
Reflecting on my decade of pioneering AI in life sciences, I’m reminded of the many challenges we faced along the way. When we first introduced AI-driven recommendations, we realized its success depended on more than just intelligence. Initial resistance from field teams, stemming from concerns over loss of control and skepticism toward unclear recommendations, highlighted a critical truth: AI solutions must empower, not replace, human judgment.
Today, I’m proud to see this philosophy drive Aktana’s Agentic AI solution. Recent months (and weeks) have brought tremendous advances in AI, and major industry announcements such as Veeva AI, Salesforce Agentforce’s support for MCP, and the Life Sciences Cloud digital labor and data acceleration partnerships. With growing willingness to embrace intelligent automation, the moment is finally right for the industry to realize the vision we set out a decade ago—and unlock its full value.
This vision is that Agentic AI has the potential to transform life sciences by streamlining operations and orchestrating omnichannel engagement, freeing professionals to focus more on strategy and outcomes. Consider this scenario:
- A Brand Manager reviews AI-suggested tactics based on a domain-specific knowledge base, confident each action is transparent and strategically-aligned.
- A Commercial Ops lead confirms that AI recommendations respect resource allocation brand tradeoffs and compliance rules, with safeguards for accuracy and traceability.
- A Sales Rep receives personalized HCP outreach suggestions—including compliant content—which she can quickly adjust to maintain her personal touch.
- A Medical Science Liaison prepares for HCP conversations with AI-curated clinical insights, focusing on what matters most.
- And of course the HCP benefits—accessing compliant information via field reps or even virtual agents, and easily scheduling follow-ups.
Notice that in all these scenarios, one principle holds: AI doesn’t replace people—it elevates them. None of this is about AI taking control away from the humans who make this all happen. It’s about amplifying human potential while reinforcing control, trust, and compliance.
Expert guidance is essential to ensure safeguards in Agentic AI, especially as Generative AI’s potential for errors (“hallucinations”) requires continuous human feedback. The life sciences industry requires a constant equilibrium between innovation and compliance. These principles have always been at the core of Aktana’s collaborative AI approach.
While the list of tasks AI will handle independently continues to grow, AI should support–not dictate– decisions. When humans are merely “on the loop,” they’re relegated to overseeing decisions without influence. Instead, they must be “in the loop“: central actors with AI augmenting their expertise, not marginalizing it.
At Aktana, we believe this is a defining moment. Agentic AI could cut down commercial planning and execution timelines from up to 18 months to as little as 6 months. Those kinds of gains — whether in faster product launches, smarter resource use, or more personalized HCP engagement—are transformative. But they’re only achievable if AI is trusted and adopted by your teams. This, in turn, requires AI solutions that are intuitive, explainable, and clearly aligned with strategic goals—all hallmarks of a human-centered AI approach.
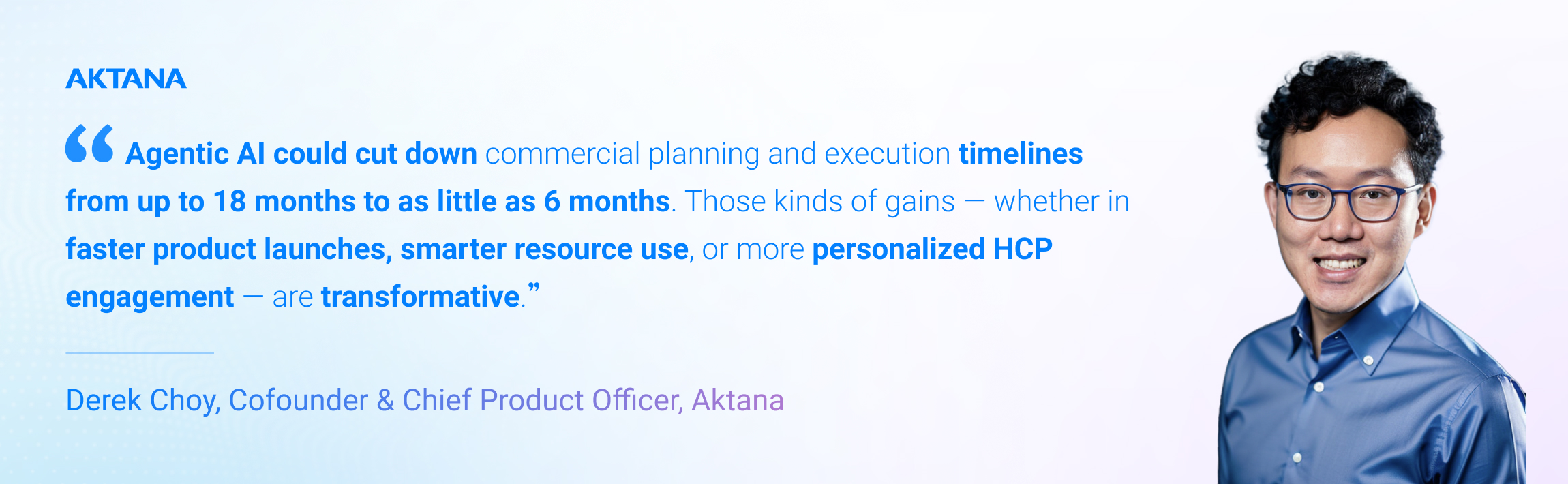
To put these insights into perspective, the section below addresses the most important questions we hear about Agentic AI in life sciences, and how to adopt it practically, transparently, and with confidence.
Frequently Asked Questions on Agentic AI
Agentic AI goes beyond analyzing data and providing recommendations—it takes action. It plans, orchestrates, and executes tasks autonomously, adapting to real-time changes. With the introduction of Model Context Protocol (MCP), it can also plug into enterprise tools and data. Unlike traditional AI, which requires defined data, predefined rules or algorithms, and requires human intervention, Agentic AI dynamically optimizes workflows while maintaining human oversight.
A useful analogy is the difference between GPS navigation and a self-driving car. Conventional AI is like GPS—it suggests the best route but leaves execution to the driver. Agentic AI, like a self-driving car, adjusts to real-world conditions, ensuring continuous optimization.
While Agentic AI introduces automation, fully autonomous AI—especially LLM-driven agents—has limitations in regulated industries. Generative AI systems are prone to hallucinations (plausible-sounding but incorrect outputs) and can drift outside of approved content or data if not constrained. A structured approach ensures AI supports decision-making without sacrificing reliability, transparency, or strategic alignment.
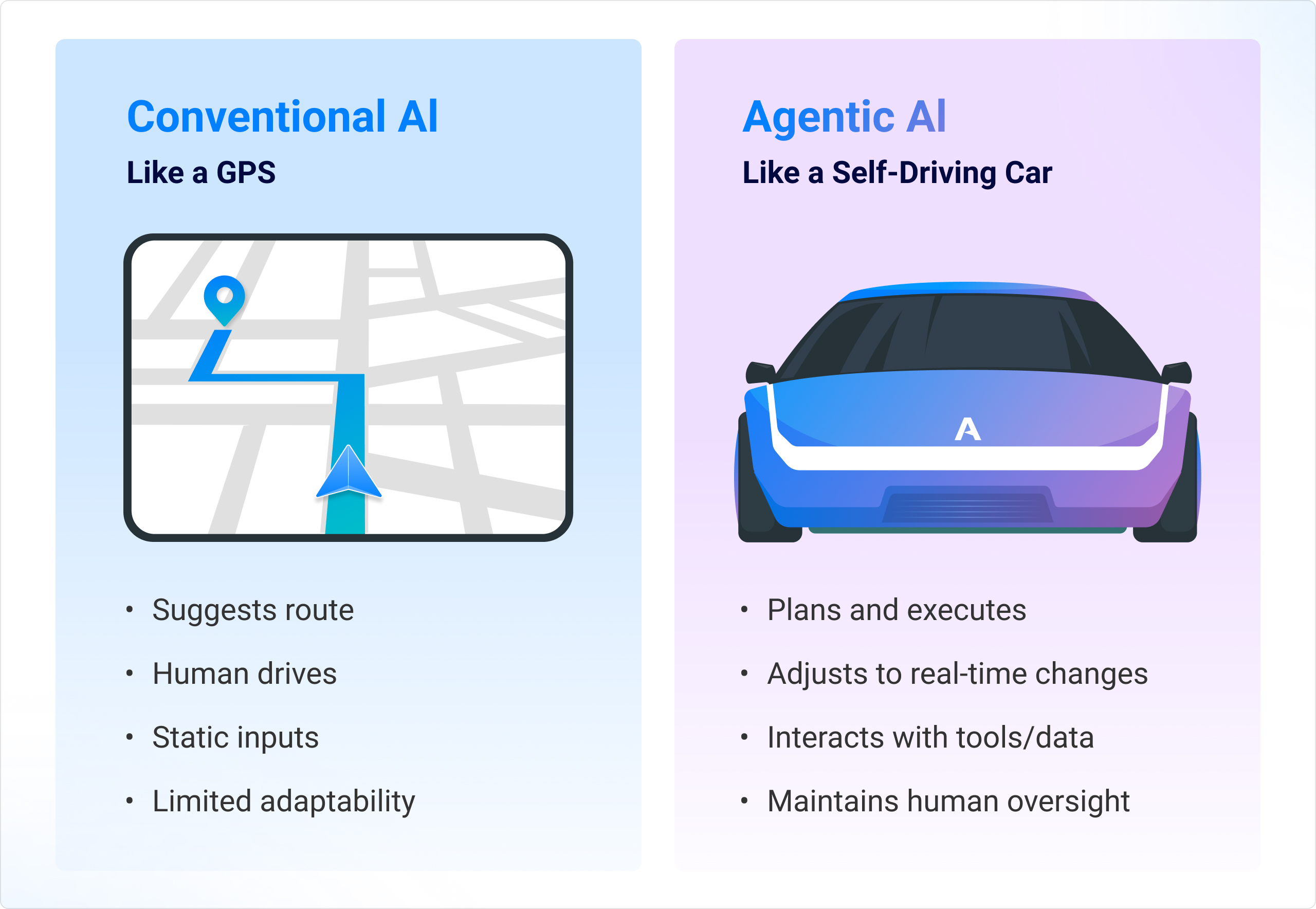
In Agentic AI systems, “agents” can be described as specialized In Agentic AI systems, “agents” are like specialized domain experts working together toward a common goal. Each has a clearly defined role, aligned to a strategic objective, and contributes to the overall decision-making process.
Think of Agentic AI as a team of intelligent specialists, modeled on how life sciences teams already operate:
- Strategy Planners define brand-level goals and KPIs to guide downstream decisions.
- Tactic Planners translate strategy into personalized HCP-level actions—what to say, when, and through which channel.
- Engagement Planners coordinate across brands and teams to align resources and avoid overlap.
- Central Optimizers, most critically, work with a Knowledge base and Orchestrators to sequence actions based on value, constraints, and compliance rules.
- Field Action Agents deliver timely suggestions and insights to reps and MSLs to support execution.
- Strategy Refiners track outcomes and KPIs to improve future recommendations.
This distributed model makes Agentic AI systems more adaptable, scalable, and transparent than single-model AI approaches—especially in the high-stakes, fast-moving environment of life sciences.
As the ecosystem evolves, standards like the Model Context Protocol (MCP) and Agent-to-Agent (A2A) communication are emerging to enable seamless interaction between agents and enterprise tools. These innovations will make it easier to extend capabilities, improve interoperability, and accelerate the adoption of Agentic AI across teams and technologies.
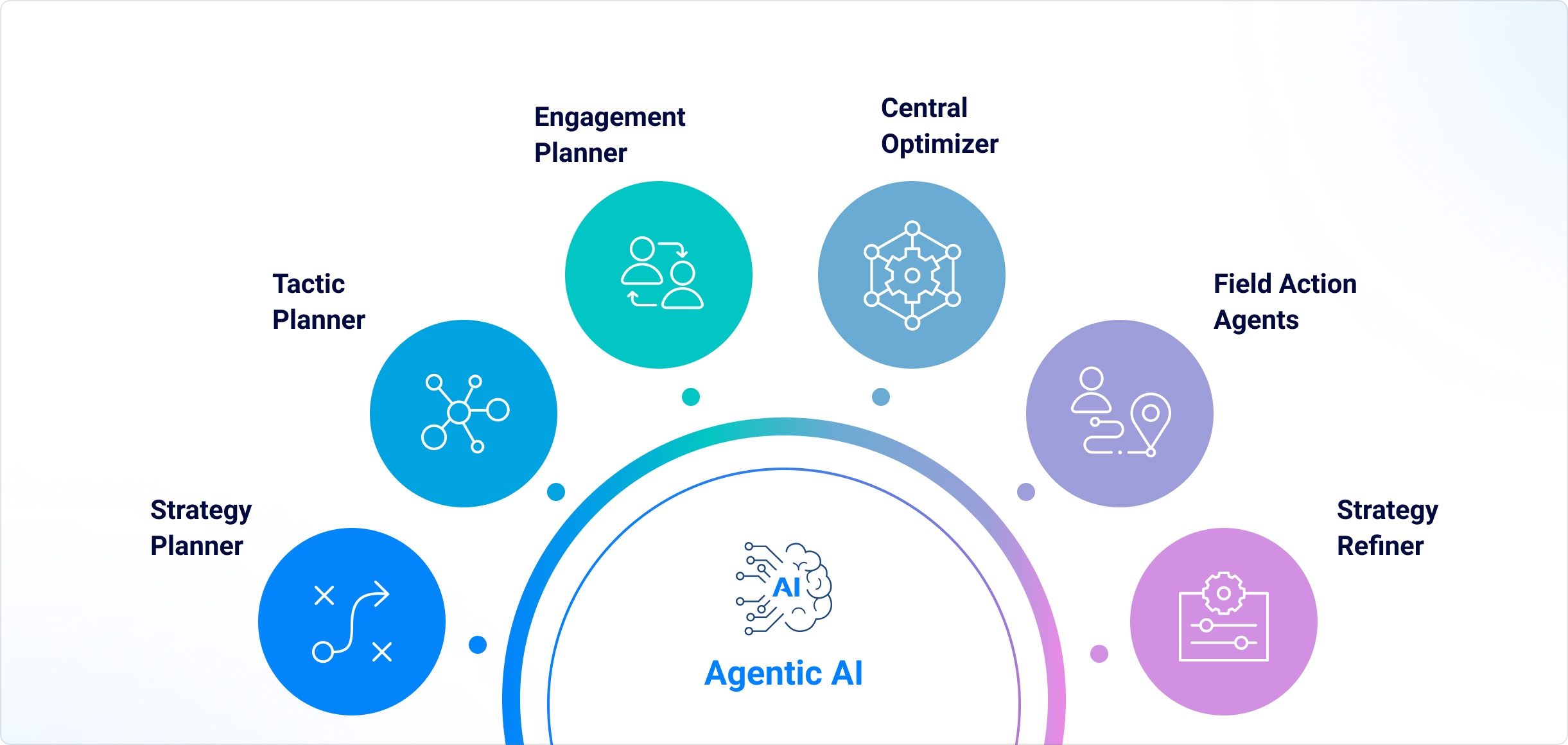
While the terms sound similar, there’s a key difference between Agentic While the terms sound similar, there’s a key difference between Agentic AI and AI agents.
- AI agents are individual specialists. Each agent performs a specific task. In life sciences, an AI agent might analyze patient data for clinical trials or generate email content for HCP engagement. These agents operate within a limited scope and often rely on external triggers or human intervention to take action.
- Agentic AI is the entire system—a coordinated network of AI agents working together to plan, execute, and optimize complex workflows. For example, in a drug launch, Agentic AI orchestrates multiple AI agents to align engagement strategies, ensure compliance, and adapt to real-time market dynamics.
Agentic AI represents a higher level of coordination and adaptability, allowing AI-driven processes to function more dynamically than individual AI agents working in isolation.
AI agents are intelligent entities that can make decisions and take action, but how they work together determines their effectiveness.
- Single-agent AI operates independently, like a solo expert tackling a project. While effective for specific tasks, a single agent lacks the ability to coordinate across complex workflows and may struggle with dynamic, multi-step decision-making.
- Multi-agent AI functions as a team of specialists, with different agents handling distinct responsibilities. They communicate, share information, and collaborate to solve problems in parallel, leading to more efficient, adaptable, and scalable decision-making.
Multi-agent AI enables specialization, coordination, and adaptability, making it ideal for complex, regulated industries. In life sciences, for example, one agent can monitor compliance, another can optimize omnichannel engagement, and others can adjust field execution based on real-time data. If one agent encounters an issue, others can compensate, ensuring continuity and resilience.
By distributing intelligence across multiple AI agents, organizations gain a system that is more flexible, scalable, and capable of handling real-world complexities without sacrificing compliance or efficiency.
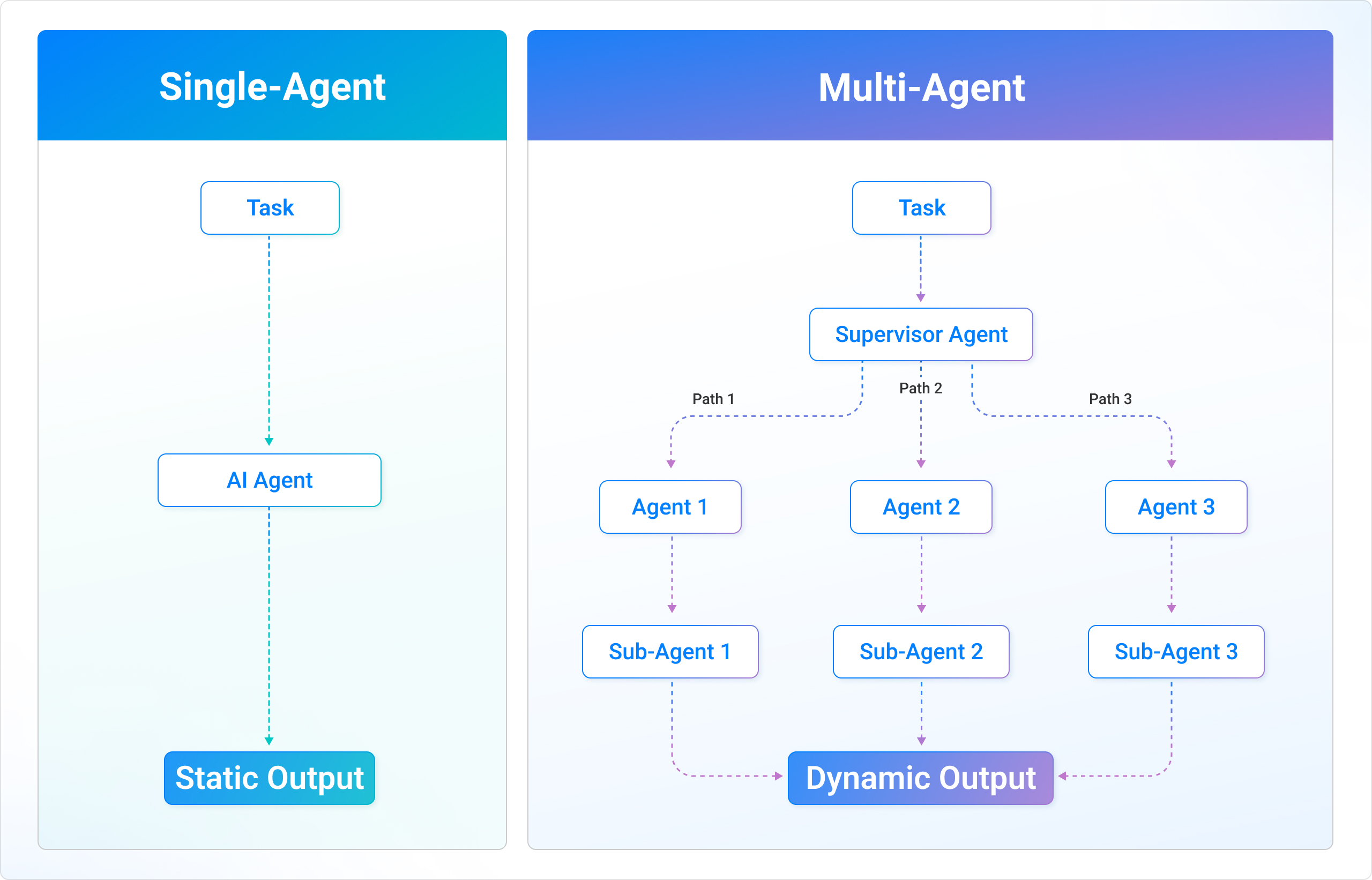
CRM and Marketing Automation systems are essential for managing HCP engagement, but they follow rigid workflows that struggle to adapt While CRM and marketing automation systems remain essential for managing HCP engagement, there is room for greater efficiency in how they are used. At present, manual intervention is often required for decision-making, adjusting strategies, and resolving errors. An AI-powered agentic system enhances these platforms by making them more dynamic, responsive, and intelligent, without disrupting existing workflows. This is made possible through a few core capabilities that define how agentic AI systems enhance CRM and marketing automation:
- Autonomous Planning – AI determines the best sequence of steps based on goals, real-time HCP behavior, and market conditions rather than relying on static workflows.
- Adaptive Execution – When prescription trends, regulatory requirements, or engagement patterns shift, AI adjusts strategies automatically to maintain relevance and compliance.
- Tool Selection and Use – AI agents interact with CRM, content platforms, and analytics tools as needed, reducing integration complexity and ensuring seamless execution.
- Memory and Context Management – AI retains engagement history across channels, ensuring continuity. If an HCP moves from digital to in-person interactions, AI adapts the outreach accordingly.
- Feedback Integration – AI refines strategies in real time, prioritizing tactics based on engagement success. If oncologists engage more with a specific content format, AI optimizes recommendations accordingly.
For life sciences companies, the goal isn’t just automation—it’s making sure AI-driven engagement works within real-world workflows, aligns with strategic priorities, and meets regulatory standards. A multi-agent approach with human oversight can support this by keeping AI adaptable, transparent, and trusted by teams.
Scaling HCP engagement is like planning a mountain climb. Traditional engagement strategies follow a fixed path based on historical data, but the real world doesn’t work that way. Competitor activity shifts, regulations evolve, and HCP behaviors change. Without real-time adjustments, teams either stick to outdated plans or scramble to adapt manually.
Just like an experienced guide adjusts a route based on conditions, Agentic AI continuously refines engagement strategies in real time. Instead of requiring teams to manually reassess every step, more advanced AI optimizes outreach, messaging, and engagement tactics to stay aligned with market realities.
But even the best AI-driven systems aren’t meant to navigate the path alone. A human-in-the-loop approach ensures that AI adapts to changing conditions while keeping commercial teams in control. Rather than replacing human judgment, this approach ensures teams can act on AI-driven recommendations with confidence, keeping personalization scalable and manageable.
General AI models are broad and adaptable, but they lack the deep industry knowledge, regulatory awareness, and real-time adaptability required in life sciences. They provide insights but rely on human intervention for execution and must be retrained to incorporate new data.
Domain-specific Agentic AI is built for complex, highly regulated environments like biopharma. It integrates compliance requirements, understands industry-specific challenges, and continuously adapts without retraining. By breaking down complex problems into smaller, testable steps, it reduces errors, ensures accuracy, and enhances decision-making, critical for regulatory adherence and commercial execution.
In life sciences, where precision and compliance are essential, AI must do more than automate tasks. It needs to support decision-making in a way that is transparent, traceable, adaptable, and aligned with business objectives.
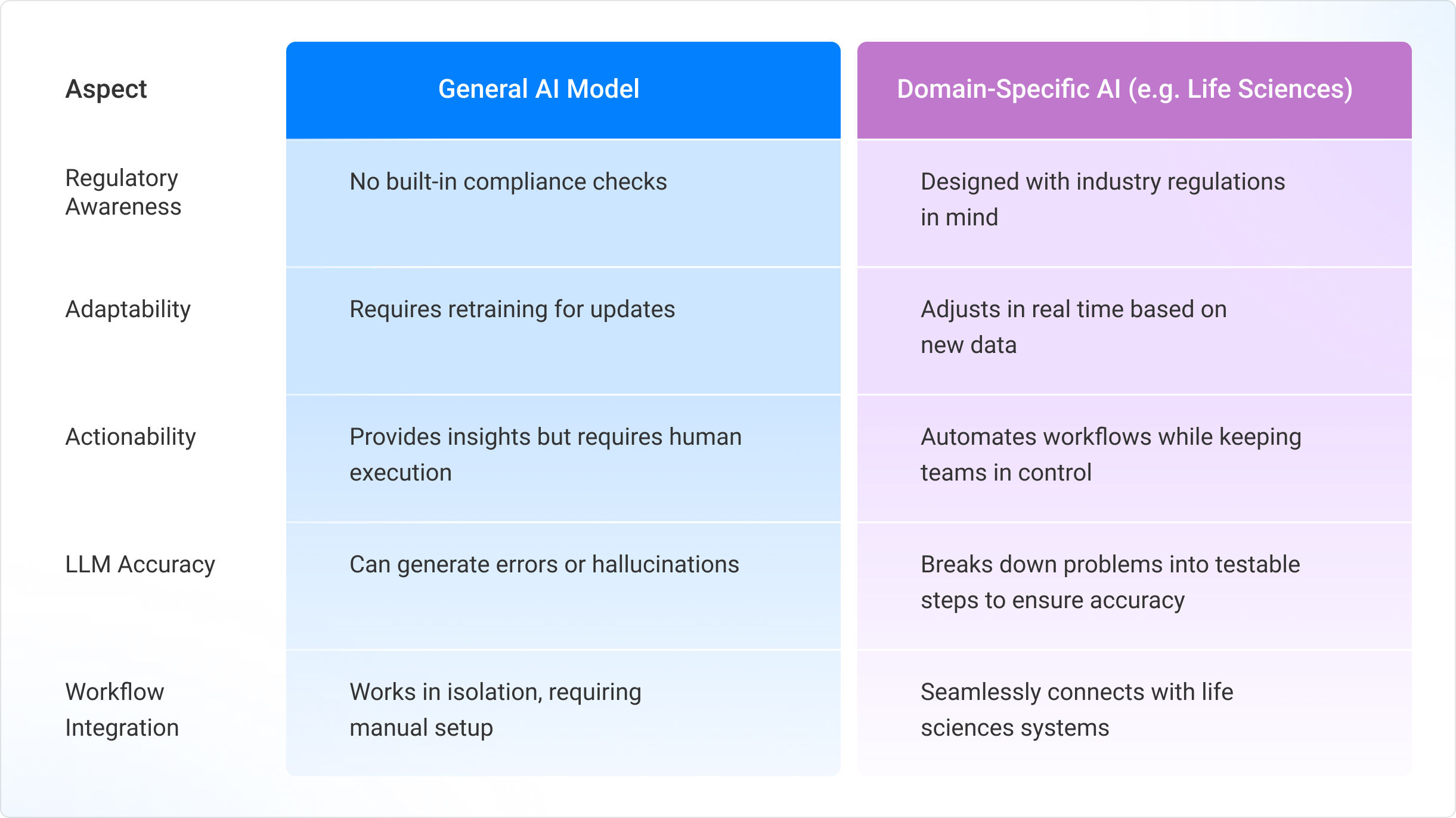
Life sciences organizations rely on CRMs, Marketing Automation, and analytics platforms to manage HCP engagement and commercial execution. The challenge is that these systems often operate in silos, requiring manual coordination and struggling to adapt to real-world complexities. Agentic AI enhances these tools by working as an intelligent layer on top of existing infrastructure, enabling real-time orchestration and adaptive decision-making.
Instead of following static workflows, AI agents analyze live data, market conditions, and HCP behavior to refine engagement strategies on the fly. It ensures that sales reps, MSLs, and marketing teams receive coordinated, context-aware recommendations, improving efficiency without increasing complexity.
This orchestration isn’t tied to a single system. Flexible integration ensures AI-driven decision-making works across platforms, rather than being restricted to a specific CRM or marketing automation tool. Companies like Aktana facilitate this through solutions that allow AI to interact with multiple systems—including Salesforce, Veeva, Adobe Experience Manager, and OCE—so organizations can apply AI-driven insights within their existing workflows.
By embedding intelligence into the omnichannel ecosystem, Agentic AI transforms CRMs and marketing automation from static execution tools into dynamic, adaptive platforms. This allows commercial and medical teams to scale engagement efficiently, ensuring that every action is optimized for real-time market conditions.
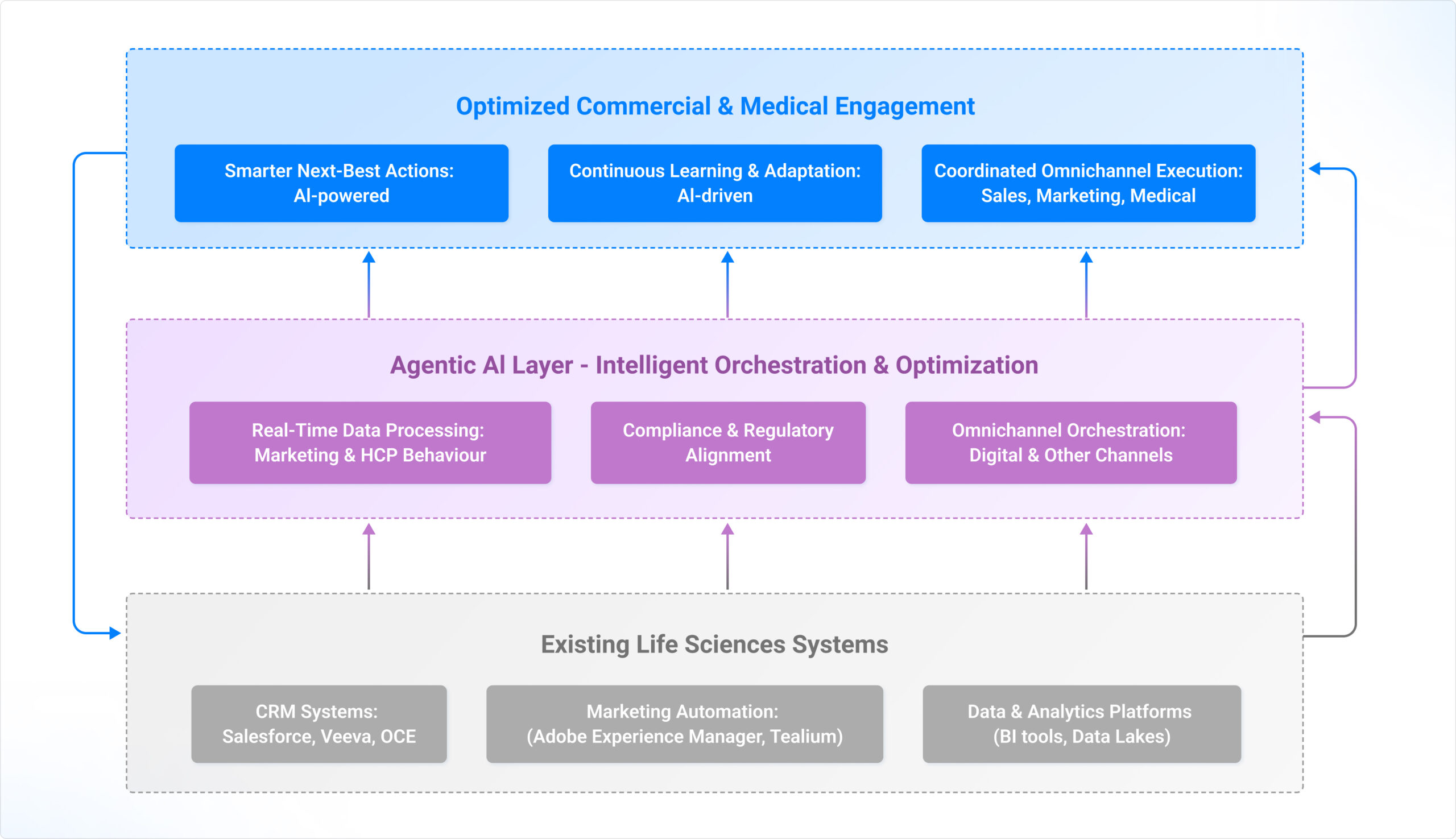
AI-driven agents in life sciences operate within a human-in-the-loop framework, balancing automation with oversight to ensure aAI-driven agents in life sciences should operate within a human-in-the-loop framework, balancing automation with oversight to ensure adaptability, compliance, and strategic alignment. Rather than replacing human decision-making, Agentic AI refines engagement strategies in real time, adjusting based on new data and expert input.
This adaptability is driven by key capabilities, including:
- Real-Time Adjustments – Teams can override AI-driven recommendations to stay aligned with market conditions and compliance needs.
- Context Retention – AI learns from human input, refining future recommendations without repeating past mistakes.
- Adaptive Workflows – AI integrates feedback continuously, adjusting strategies based on HCP behavior, rep interactions, and regulatory updates.
- Strategic Oversight – AI supports, rather than replaces, human decision-making, ensuring engagement remains effective and compliant.
But these alone aren’t enough. AI needs both structure and context to be effective in life sciences. Without these, black-box AI risks making opaque, misaligned decisions that introduce inefficiencies or compliance risks.
- AI needs a central intelligence layer to orchestrate decisions – To align execution with business goals, AI must continuously weigh competing priorities, optimize actions across channels, and integrate constraints like compliance and resource availability.
- AI must be guided by contextual knowledge – Industry expertise, historical data, and regulatory frameworks provide the foundation AI needs to make informed, transparent, and strategically sound decisions.
Aktana enables this by ensuring AI-driven execution is not just automated, but adaptive, explainable, and aligned with real-world business needs.
What’s Coming Up Next
Human expertise is what makes AI valuable, but trust is what makes AI usable. In the next part of our FAQ series on Agentic AI, we’ll explore why transparency is non-negotiable in AI adoption—and how organizations can build confidence in AI-driven decision-making.
In the meantime, explore our Agentic AI page to see how these principles come to life across real-world use cases in life sciences—and why so many industry leaders are rethinking what AI can do.